Sanjana S is a student of BBA LLB, Symbiosis Law School, Hyderabad.
Introduction
In the ever-advancing landscape of healthcare, Artificial Intelligence (AI) stands as a transformative force, promising to enhance diagnostic accuracy, treatment recommendations, and patient care. As per Statista’s data, the AI healthcare sector, which had a market worth of $11 billion in 2021, is anticipated to surge significantly, reaching a staggering valuation of $187 billion by 2030. This substantial growth is indicative of the profound transformations that are poised to reshape the operational landscape of medical providers, hospitals, pharmaceutical and biotechnology firms, as well as various stakeholders within the healthcare industry. AI systems, powered by immense datasets and sophisticated algorithms, have the potential to revolutionize the medical field. However, this technological leap forward is not without its challenges, and one of the most pressing concerns is algorithmic bias. In this article, I will first initiate a comprehensive exploration into the origins and mechanisms through which algorithmic biases infiltrate healthcare AI systems. Subsequently, I will discuss the ramifications arising from the prevalence of biased healthcare AIs within the health sector, from both a social and legal perspective. Finally, I will argue for the proactive adoption of industry best practices, specifically the need for an explainable AI and the usage of synthetic data, as a strategic intervention to alleviate the pervasive issue within the healthcare sector.
Examining the causes and mechanisms underlying algorithmic bias in healthcare AI
Algorithmic bias in healthcare AI is not a hypothetical concept; it is a very real and pervasive issue that affects individuals’ lives daily. Simply put, algorithmic bias in healthcare AI refers to the prevalence of unjust and discriminatory consequences in medical decisions made by AI systems. It arises when algorithms, which are frequently trained on biased data, show inequalities in predicting or diagnosing health issues across demographic groups. Bias, whether deliberate or accidental, can infiltrate AI algorithms, resulting in inaccurate diagnoses, inequities in treatment, and possibly life-altering repercussions.
To provide additional clarity, here’s an example of algorithmic bias in healthcare AI: In October 2019, an investigation revealed that an algorithm deployed across US hospitals, assessing the likelihood of patients requiring additional medical attention for over 200 million individuals, exhibited a significant bias in favor of white patients over black patients. Although the algorithm did not explicitly consider race as a variable, it heavily relied on another factor closely associated with race, namely the medical cost history. The underlying assumption was that healthcare expenses provide a comprehensive reflection of an individual’s medical needs. Notably, due to various factors, black patients tended to have lower healthcare costs compared to white patients with similar medical conditions.
Fortunately, subsequent to scrutiny, researchers collaborated with Optum to mitigate the bias substantially, achieving an 80% reduction. This intervention was crucial in preventing the perpetuation of severe discriminatory biases within the AI system. Without the initial investigation and corrective measures, the algorithmic bias would have persisted, adversely affecting the equitable treatment of patients.
Systemic Inequities in Training Datasets for Artificial Intelligence Models
Algorithmic bias in healthcare AI stems from various underlying factors. For one, there exists the inherent challenges posed by systemic inequities embedded in societies and health systems. More specifically, the lack of contextual specificity in health systems further contributes to algorithmic bias. Varying designs, objectives, and diverse populations served by different health systems create challenges in developing a universally applicable AI model. Moreover, insufficient data (that are collected to embed into datasets used for machine learning) for underrepresented socio-economic groups result in imbalances, hindering accurate predictions for these groups.
For instance, Nicholson Price, a scholar affiliated with the University of Michigan’s Institute for Healthcare Policy and Innovation, contends in his paper titled “Exclusion Cycles: Reinforcing Disparities in Medicine” that entrenched biases against minoritized populations, contribute to self-reinforcing cycles of exclusion in healthcare. The paper emphasizes how these biases extend beyond Black patients to include other minority groups, such as Native American patients, transgender patients, individuals with certain disabilities, and even women, despite being a numerical majority. The central argument revolves around the cyclical dynamics in research participation and recruitment, extending to the realm of AI. In other words, in the majority of research studies, minorities are frequently overlooked or excluded.
In another instance, a notable example of algorithmic bias in healthcare AI diagnostic systems involves a study that found racial disparities in skin cancer diagnoses. The algorithms, trained on datasets predominantly composed of images from lighter-skinned individuals, demonstrated significantly lower accuracy in detecting skin cancer on images of darker-skinned patients. This highlights how biases in training data can lead to disparities in diagnostic accuracy across different racial groups, potentially exacerbating healthcare inequalities.
Lastly, historical healthcare biases lead to inadequate recruitment of minority groups for research studies. This insufficient engagement perpetuates the perception that minoritized patients are less interested in research, creating a reinforcing cycle of exclusion. As a result, the exclusionary practices in data collection contribute to insufficient and biased training datasets for AI systems. This inadequacy perpetuates discriminatory patterns in the predictions, classifications, and recommendations made by the AI, reinforcing existing biases in clinical care.
Black-box ML/DL Models
Black box deep learning can be described as an artificial intelligence model where the inputs and operations remain concealed from users or relevant stakeholders. These models function opaquely, arriving at medical conclusions or decisions without offering explicit insights into the underlying processes or reasoning that led to those outcomes.
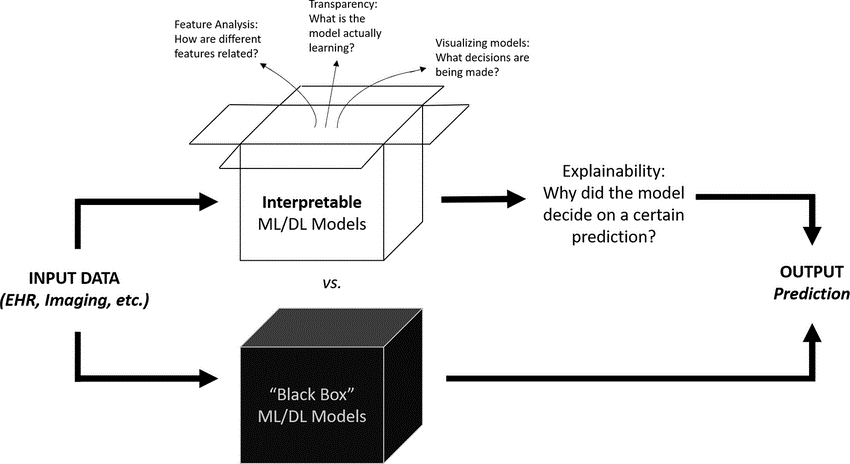
Fig 1: Interpretable ML/DL Models vs. Black Box ML/DL Models. Source: Aaron Hui, ‘Ethical Challenges of Artificial Intelligence in Health Care: A Narrative Review’.
Black-box models, characterized by their opacity, pose difficulties in identifying and rectifying biases, particularly those leading to a higher frequency of errors among patients from underrepresented or marginalized groups. This phenomenon, often termed “uncertainty bias,” has the potential to perpetuate existing healthcare inequities and exacerbate outcomes for vulnerable patients. Notably, Obermeyer et al. underscored a concerning bias where an algorithm erroneously predicted that Black patients required fewer medical resources than White patients, perpetuating historical disparities in healthcare resource allocation without an explanation per se. Thus, the inherent difficulty in avoiding bias arises from the opaque nature of black box models and imperfect training data thus leading to the challenge of ensuring representativity, richness, and accurate labeling.
Legal Implications of AI Implementation in Healthcare
The intersection of artificial intelligence and healthcare introduces a critical dimension of legal scrutiny, particularly concerning the potential misdiagnoses stemming from biased AI systems. As these systems increasingly play a role in clinical decision-making, the legal landscape will grapple with the ramifications of erroneous outcomes, addressing questions of accountability, patient rights, and the ethical implications of algorithmic bias in the healthcare domain.
As indicated in the Draft National Strategy for Artificial Intelligence, India actively welcomes AI integration in healthcare. Recognizing the potential to address long-standing healthcare challenges, collaboration between the government, tech firms like Microsoft, and healthcare providers, as exemplified by initiatives like the diabetic retinopathy early detection pilot and the International Centre for Transformational Artificial Intelligence, demonstrates the country’s commitment to leveraging technology for improved healthcare outcomes. However, due to the lack of explicit rules governing AI technology, India confronts legal issues in the AI healthcare industry. The lack of well-defined laws raises concerns regarding accountability for failures in AI-enabled medical practices. The legal ramifications and ethical considerations surrounding the use of AI in healthcare remain unknown, including questions of responsibility for errors and potential discrimination, underscoring the critical need for regulatory frameworks in this arena.
As far as the United States of America is concerned, the U.S. government, led by the Biden administration, has issued a presidential executive order establishing new guidelines and regulations for Artificial Intelligence (AI). Released on October 30, President Biden has issued a groundbreaking Executive Order, setting new standards for AI safety and security to position America at the forefront of harnessing AI’s promise while managing its risks. The order prioritizes protecting privacy, advancing equity and civil rights, advocating for consumers and workers, promoting innovation and competition, and reinforcing American leadership globally in the realm of artificial intelligence in healthcare. In the UK, the use of AI lacks specific legislation, relying instead on a set of general laws like the UK Medical Device Regulations 2002 and the Data Protection Act 2018. This absence of dedicated regulations raises challenges in addressing the nuanced implications of AI applications and underscores the need for comprehensive legal frameworks tailored to AI technology, particularly in the health sector.
The absence of robust regulations governing AI in healthcare raises significant concerns. As AI rapidly advances within this sector, it becomes imperative to establish comprehensive regulations that not only address the accountability for potential negative impacts but also ensure the development of high-quality, unbiased, and reliable AI systems. Policy frameworks must be carefully crafted to prevent and mitigate biases, fostering an environment where AI technologies contribute positively to healthcare outcomes while upholding ethical standards.
Mitigating Algorithmic Bias in Healthcare AI: Explainable AI and the usage of synthetic data
Explainable AI
Explainable AI (XAI) refers to artificial intelligence systems’ ability to provide explicit and transparent justifications for their decisions. XAI works in healthcare AI to address the complexities and lack of clarity in machine learning systems, particularly in medical contexts, by providing explanations that are understandable and reliable for medical practitioners. XAI is a solution for minimizing bias in healthcare AI by providing institutional explanations that answer medical practitioners’ concerns about the dependability and credibility of AI systems. These explanations focus on demonstrating why medical practitioners should trust the AI system, with the goal of assuaging worries about the organizations supervising medical AI design. By maintaining openness about the system’s assumptions, XAI helps to avoid unaddressed biases and errors, contributing to a more egalitarian and unbiased healthcare AI field. Furthermore, XAI aids in discovering and correcting biases in AI algorithms by providing post-hoc explanations that allow stakeholders to scrutinize the most influential aspects influencing the system’s judgements. These technical explanations are critical for stakeholders involved in specifying or optimizing the machine, allowing for the detection and correction of model biases.
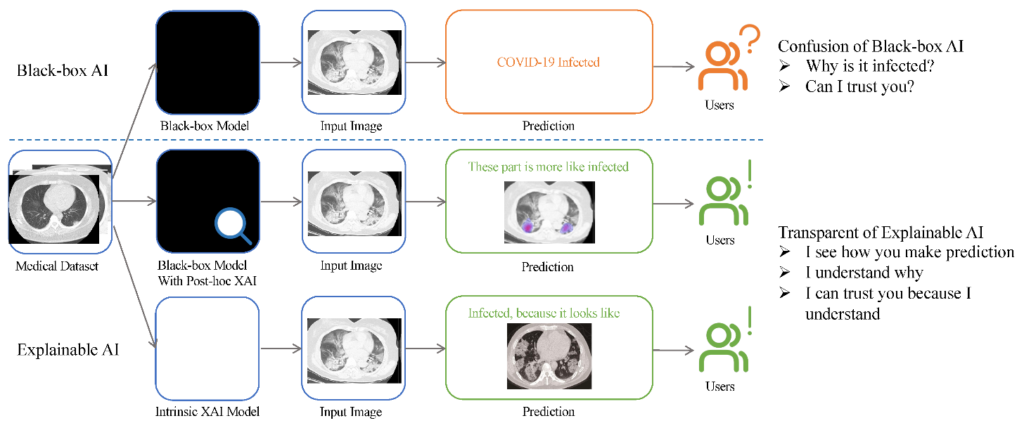
Figure 2: A visual comparison flowchart illustrates the distinctions between black-box and explainable artificial intelligence (AI) and their respective impacts on the user experience. The upper branch delineates the functioning of a black-box model, which typically furnishes outcomes in the form of classes, such as identifying whether an image pertains to COVID or non-COVID. Contrarily, the middle and bottom branches signify two explainable AI (XAI) approaches, showcasing alternative methods that offer transparency and insights into the decision-making process. Source: Ahmad Chaddad, ‘Survey of Explainable AI Techniques in Healthcare’.
Synthetic data
In machine learning for healthcare AI, synthetic data refers to artificially generated data that mimics real-world patient information but is not derived directly from actual persons. This artificially produced dataset is intended to retain the statistical features and patterns of genuine patient data, allowing machine learning models to be trained and improved without jeopardizing patient privacy or introducing biases from the original data sources. In healthcare AI, synthetic data is especially useful for tackling difficulties such as data scarcity, privacy concerns, and bias mitigation during AI model constructions. Synthetic data can aid in reducing AI bias in healthcare by diversifying training datasets, mitigating the impact of skewed or underrepresented patient populations and fostering more equitable and accurate AI models.
Conclusion
Artificial Intelligence (AI) is poised to be a revolutionary force in healthcare, promising advancements in diagnosis, treatment recommendations, and patient care. However, this transformative journey encounters challenges, notably algorithmic bias. This article has first delved into the origins and mechanisms of algorithmic bias in healthcare AI, exploring its societal, legal, and ethical implications. Proactive adoption of best practices, including Explainable AI models and the integration of synthetic data, is pivotal to address challenges in the AI healthcare sector and mitigate biases effectively. The absence of robust legislation governing AI in healthcare underscores the necessity for comprehensive frameworks, ensuring the development of unbiased, reliable, and high-quality AI systems. By leveraging best practices, such as Explainable AI and synthetic data, there is promising potential to mitigate biases, fostering an environment where AI contributes positively to healthcare outcomes while upholding ethical standards.